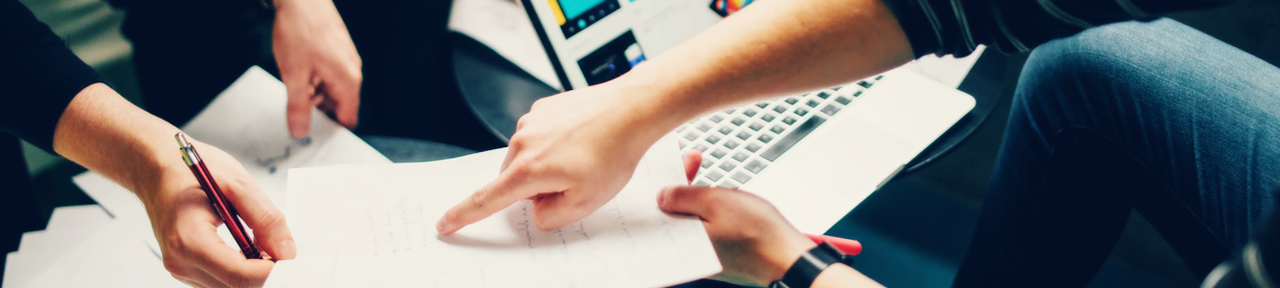
Traditional statistics primarily focuses on theoretical research related to probability and various statistical issues such as random variables, sampling distributions, hypothesis testing, estimation theory, large sample methods, regression analysis, and statistical modeling. Many universities have established theoretical-oriented statistical research institutes with these topics as their main development goals. However, the urgent practical needs of real-world problems stimulate new developments in statistics, such as engineering statistics, financial statistics, and one of the current major research areas in statistics, Health Data Analytics and Statistics. Health Data Analytics and Statistics is mainly driven by practical challenges faced by medical and public health research institutes, stimulating developments in the field of statistics. The main issues currently addressed by Health Data Analytics and Statistics deserve in-depth research, improvement, and further development.
1. Omics Data Analysis and Statistics
Since the completion of the Human Genome Project (HGP), various omics studies have flourished, including genomics, epigenomics, transcriptomics, proteomics, metabolomics, microbiomics, exposomics, and more. Omics data are characterized by large p and small n (many variables but few samples), necessitating the development of specialized statistical methods. These include efficient multiple testing corrections, methods to enhance power through aggregating numerous variables, and bioinformatics algorithms considering gene regulation and gene networks. Omics data are diverse and often originate from different platforms, posing challenges for integrated analysis in statistical methodology.
Omics data analytics not only focuses on cross-omics, cross-species, and cross-platform studies but also emphasizes interdisciplinary collaboration. In addition to statistical, mathematical, and informatics approaches, omics data analytics must integrate dry lab methods with wet lab methods from life sciences and basic medicine (such as cell biology and molecular biology). This integration is essential for exploring functions, mechanisms, regulations, and human health effects, thereby making significant scientific contributions.
2. Health Data Topology Statistics
Health big data, such as global burden of disease data, cancer registry data, national health insurance data, and environmental monitoring data (e.g., air pollution, soil heavy metal contamination), all require further development of appropriate statistical methods. For instance, age-period-cohort models are used to analyze long-term trends and future predictions of disease incidence and mortality rates. Population-based cancer survival studies utilize relative survival analysis to overcome misclassification of causes of death. Propensity score methods are employed in national health insurance research to adjust for unmeasured confounding factors. Methods to overcome immortal time bias in study designs and statistical analyses are also critical. Environmental monitoring data necessitate the development of spatiotemporal models, integrating time series analysis and spatial analyses such as kriging and land-use regression.
3. Health Internet of Things (HIoT) Statistics
The Internet of Things in healthcare (HIoT) is poised to revolutionize healthcare delivery. HIoT integrates electronic health records, medical imaging data, mobile health data, wearable device health monitoring data, home monitoring data, and more. There is a pressing need for the development of appropriate statistical methods to harness this wealth of data effectively.
Methods such as text mining, tensor-based learning, dimension reduction techniques, deep learning, and recommender systems, which combine artificial intelligence with statistical approaches, are crucial. Statistical analysis in HIoT also requires leveraging cloud computing technologies and network analytics methods.
These advancements depend on interdisciplinary collaboration across statistics, mathematics, informatics, biomedical engineering, clinical medicine, and public health. They aim to enhance the quality of telemedicine and home care, integrate resources, and reduce the burden on physical healthcare facilities.
4. Etiology and pathogenesis research methods
Chronic diseases such as cancer, cardiovascular diseases, and others are often complex in their etiology, involving intricate relationships of synergy, antagonism, and mediation among numerous genetic and environmental factors. The presence of unknown or unmeasured factors can pose significant challenges in clarifying causative pathways and disease mechanisms.
Statistical methods for causal inference, such as counterfactual potential outcome models, causal-pie models, structural equation models, and Mendelian randomization methods, have made notable advances in recent years towards understanding disease causation and pathogenesis.
5. Natural history of disease, screening, diagnosis and prognosis research methods
Chronic diseases, such as cancer, encompass a natural history that spans from the generation of cancer cells through symptom onset, diagnosis, treatment, recovery, disability, and ultimately death. Screening for diseases targets detectable preclinical periods, but variability in the duration of these periods introduces biases such as lead-time bias and length-time bias. Specialized statistical methods are required to address these issues.
The evaluation of screening effectiveness relies on advanced statistical models such as Markov transition models and multistage carcinogenesis models. Evaluation of diagnostic tools goes beyond traditional measures like sensitivity and specificity, also rely on statistical methods such as receiver operating characteristic (ROC) curve analysis and decision curve analysis. In terms of disease prognosis, there is a need for developing statistical methods such as regression trees for survival data and disability-adjusted survival analysis.
6. Mathematical model of infectious diseases
The COVID-19 pandemic has caused immense impacts on public health, society, and the economy. Analysis of infectious disease data such as epidemic curves, spot maps, contact tracing, and social networks can initially elucidate the epidemiological characteristics of the disease. Mathematical models of infectious diseases, such as the susceptible-exposed-infected-recovery (SEIR) model, agent-based modeling, and others, can predict potential epidemic patterns and evaluate the effectiveness of various control measures (such as social distancing, contact tracing, isolation, and lockdowns).
7. Observational research methods in clinical medicine and public health
Observational studies are commonly used research designs in clinical medicine and public health. Observational study designs such as cohort studies, case-control studies, case-only studies, case-cohort studies, case-parents studies, twin studies, family studies, pedigree studies, and others are prone to various biases such as selection bias, information bias, and confounding bias. It is essential for researchers to continue developing statistical methods for study design and data analysis to mitigate these biases and improve the quality of observational research.
8. Clinical trials and evaluation of public health intervention programs
Clinical trials provide rigorous evaluation of healthcare interventions. Phases I to IV clinical trials involve specialized statistical methods including dose finding, sample size calculation, randomization methods, control selection, and others, which warrant in-depth exploration. Public health intervention programs often cannot utilize randomized assignment for evaluation. Therefore, they require special statistical methods such as difference-in-difference studies, interrupted time series analysis, and others.
9. Integrated medical and public health analyses and decision-making analyses
Meta-analysis and network meta-analysis consolidate findings from multiple studies, forming the foundation for evidence-based and precision medicine/public health. Decision analysis and network decision analysis weigh cost and benefits, providing guidance for formulating clinical guidelines and public health policies. The statistical methods of meta-analysis and decision analysis deserve further research. Moreover, Bayesian statistics plays a crucial role across various domains of health data analytics and statistics, warranting vigorous promotion and development.